Legal Apocalypse?
An In-House Counsel Parses AI
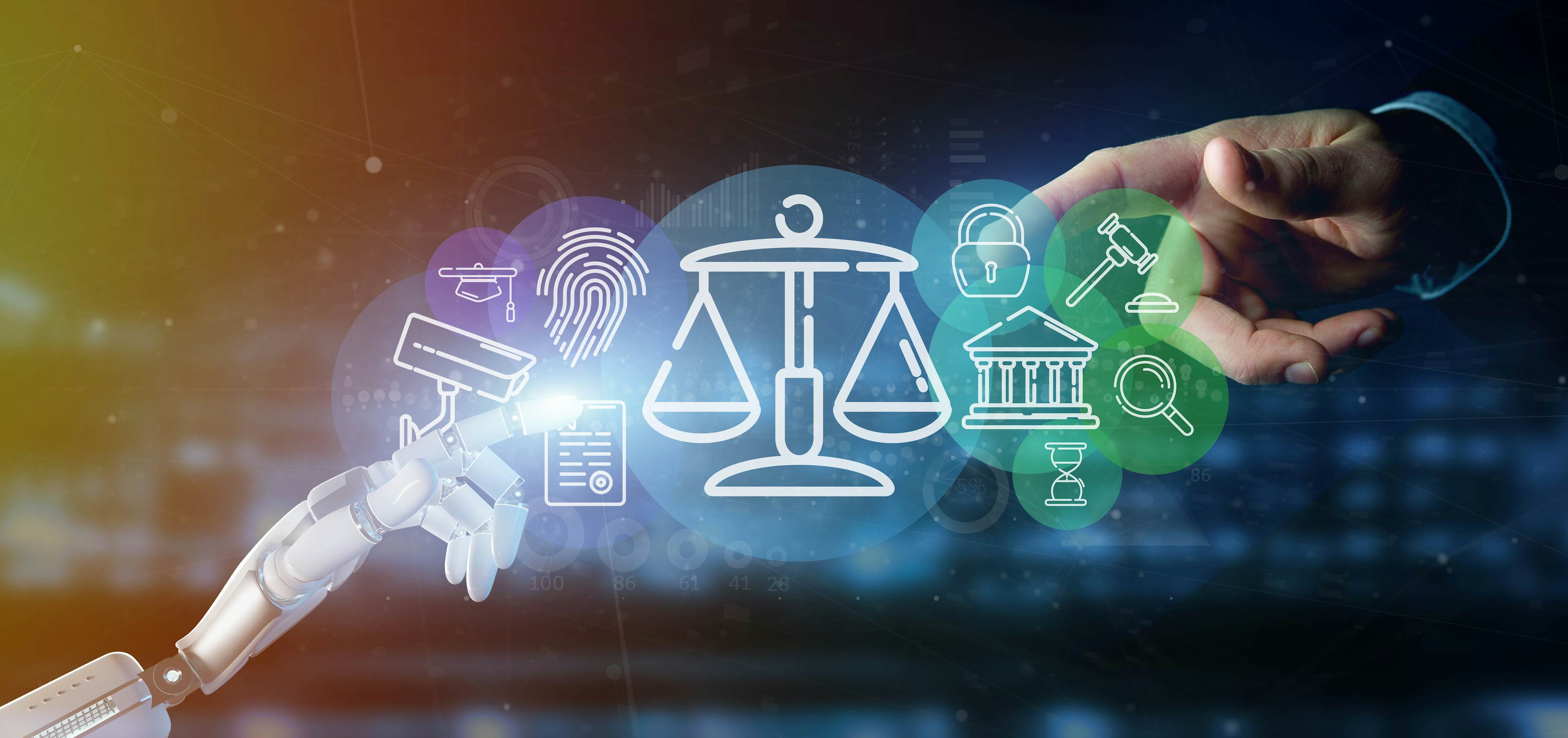
‘Legal Apocalypse? An In-House Counsel’s Parses AI’ delves into the impact of generative AI on the legal profession. It assesses which legal practices and positions are more likely to face disruption and those relatively safe from AI influence. The blog navigates the spectrum of AI’s problem-solving capabilities, from well-defined to ill-defined problems, and scrutinizes the potential transformations in various legal domains — including litigation, document review, legal analysis, and transactional work. The article concludes with a perspective on how AI’s evolution might shape legal practices and the importance of integrating AI thoughtfully into the legal field.
The advent of an extinction-level event for the legal profession appears to be upon us. “Law” has consistently ranked high on the leaderboards of fields poised to be disrupted by Generative AI. But, as the profession so often says, “It depends.” Not all legal practice areas are created equal, and not all lawyer subspecialties face the same risk from GPT applications. In this article, I’ll discuss my outlook on which areas of law are most likely to be disrupted by artificial intelligence and which appear to be safe – perhaps until the release of GPT-5.

Chris Browne
Deputy General CounselChris is an in-house attorney for Alumni Ventures, a national venture capital firm focusing on AI. He has been a judicial clerk at trial and appellate levels, an adjunct professor of law, in-house counsel to a prominent national registered investment advisor, senior counsel to a well-known litigation boutique, an arbitrator for FINRA, and a solo practitioner providing advice and representation to hedge funds and other financial institutions focused on private investments.
How Intelligent Is Artificial Intelligence?
At least until the advent of ChatGPT, when most people heard the term “artificial intelligence,” they probably envisioned HAL, Roy Batty, or Agent Smith from popular entertainment. A close simulation of human “general intelligence,” widely adaptable to any circumstance, able to tackle any problems, and often accompanied by a personality resembling that of an adult human being. The reality of AI, as it exists today, is something different.
Let’s begin by saying that the basic meaning of “intelligence” for the purposes of this blog post is the ability to solve problems. If we define intelligence this way, we’ve been working on steadily more sophisticated forms of AI for the better part of a century already. Starting in the 1960s, Texas Instruments offered a pocket calculator capable of solving basic arithmetic problems. In the 1980s, Grammatik offered the first computer program to check English grammar. In the 1990s, Norton first released its antivirus software program. And throughout the 21st century, a number of businesses have developed and released progressively more sophisticated chatbots from SmarterChild to Siri to ChatGPT-4.
Throughout this evolution, the problem-solving capabilities of AI have moved along a continuum from Well-Defined Problems to Ill-Defined Problems. A Well-Defined Problem is narrow in scope, has only one answer, and can usually be solved with a small universe of inputs. An Ill-Defined Problem is open-ended, has multiple solutions, depends on a wide variety of inputs, and does not always have a clear best answer.
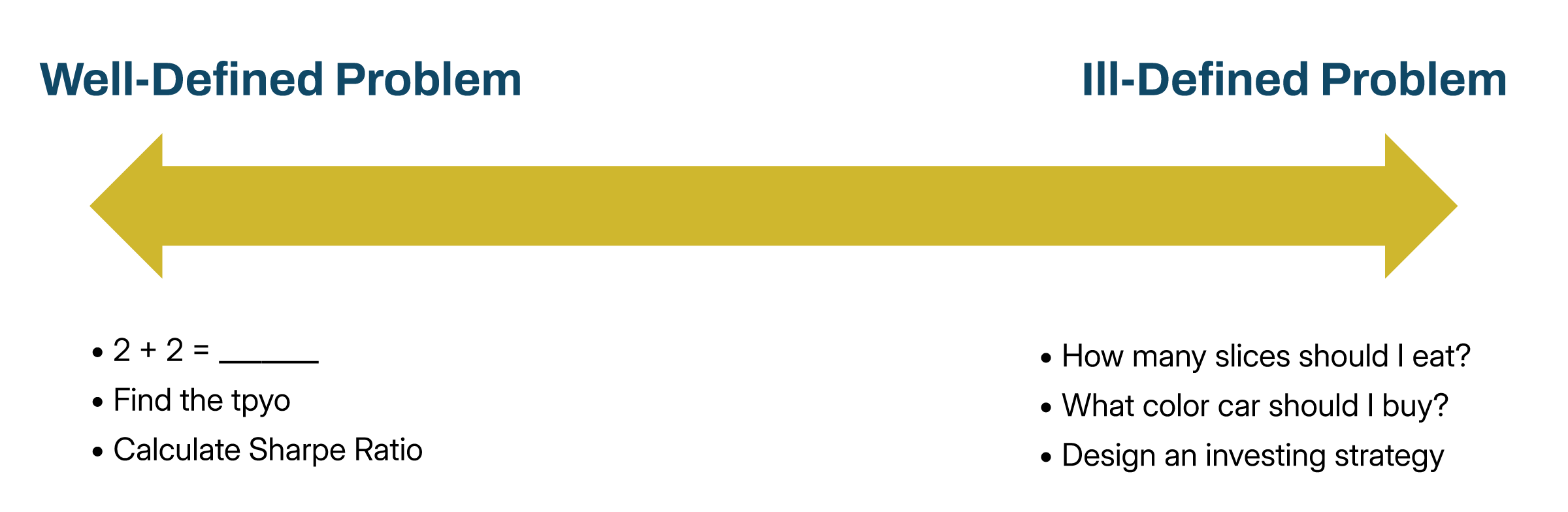
In other words, what we’ve been seeing with the breakout of ChatGPT and related AI modules is the problem-solving ability of AI moving from left to right along the spectrum above. No longer are computer programs limited to applying static algorithms to fixed inputs to derive a singular answer. A freshman in high school at the turn of century might have asked their iMac’s local software to grammar-check their essay on George Washington. A freshman in high school today can log into ChatGPT to ask it to write the essay from scratch.
That being said, AI has not yet successfully navigated all the way to the edge of the Ill-Defined Problem side of the spectrum above. First, while you can ask AI to write a school essay, hardware and processing constraints limit its ability to produce a novel or a movie script for you.
Second, in my experience, AI struggles noticeably in differentiating high-quality inputs from low-quality inputs on a given subject. So in using an AI to research complex or broad topics, you may get a result that incorporates incorrect information, misconstrues some of the nuances of the subject, or glosses over important details.
And third, AI can’t yet navigate problems past a certain level of subjectivity. While you could probably train an AI on a loved one’s preferences so it could provide good holiday gift ideas, concepts like what terms of a merger deal are “fair” or what kinds of marketing could “cause a misleading inference to be drawn” depend too much on a uniquely human way of seeing the world and making moral judgments for an AI to be useful.
Finally, it’s important to keep sight of how AI works. Through natural language processing, AI can extrapolate patterns from large volumes of text to guess what language comes next in a given sequence. This allows AI to credibly interact with human users through human language.
But this ability doesn’t allow AI to substantively understand the words it reads and writes. Suppose our high school freshman asks Chat-GPT, “Was George Washington an effective military commander in the early years of the Revolutionary War?” The AI has no understanding of who George Washington was, and can’t form opinions about his effectiveness. The only thing it can do is match the terms of the student’s question with other text, cobble together something that matches the patterns of human speech used to train it, and offer that as a response.
The Practice(s) of Law
As anyone who’s navigated the legal job market knows, the practice of law isn’t a monolith. From the day budding summer associates are sorted into Litigation or Transactional work, every new lawyer gradually settles into a distinct niche. Macroeconomic developments, market conditions, and exogenous disruptions can have profoundly different effects on lawyers, depending on their area of practice. The Great Recession was devastating for M&A activity, but a boom time for bankruptcy specialists. The Covid pandemic dealt commercial real estate a blow from which it still hasn’t fully recovered, but healthcare and data privacy law saw growth through those years of Zoom meetings and social distancing. Unsurprisingly then, the advancement of AI means different things for different practices.
Litigation
Litigation involves a range of problems to solve, some of them Well-Defined and some of them Ill-Defined. I generally see a larger, more disruptive role for AI where litigation work incorporates Well-Defined Problems, while the work of solving Ill-Defined Problems looks to remain relatively insulated from significant disruption. Breaking down the Litigation sector roughly along the lifecycle of a given court case, we have the following.
Legal Research Resources
From the familiar stalwarts of Westlaw and Lexis to the new challengers of Fastcase and Bloomberg, the legal research sector is poised for major disruption by AI. The primary reason for this vulnerability is price. While the days of hourly billing for Westlaw and Lexis that sometimes invited comparisons to hourly charges for private jet flights have long ended, most legal research services remain significant cost centers for attorneys, with some plans approaching or exceeding $1,000 per month, per account. Compare that cost to the $25 per month for OpenAI team pricing as a potential baseline comparison.
In addition, natural language processing is likely to simplify the process of searching databases of legal authorities into something more accessible and less dependent on specialized search vocabulary, changing something like this:
(“false claims” /s retal! /s “but for”) & CT(CA1)
Into this:
Provide excerpts of all decisions of the U.S. Court of Appeals for the First Circuit that discuss the but-for causation standard governing retaliation claims under the False Claims Act.
But that’s only the beginning. Natural language processing can do more than parse voluminous legal authorities for relevant passages. It can review opposing filings and prompt itself to execute research tasks to locate adverse authorities, factual distinctions, and contrary evidence in an exhibit binder, or even a Relativity environment.
While big changes are coming to the world of legal research, there will probably still be a place for incumbents. If nothing else, incumbents’ ability to obtain publications of primary and secondary authorities means that a hypothetical AI-based legal research service may need to rely on one of the incumbents to get hold of authorities that aren’t widely published, like lower state courts or industry arbitrators, for integration into a database supporting an AI. Moreover, there’s probably something to be said for first-mover advantage in allowing Westlaw or Lexis to develop their own legal research AI, if it can remain price-competitive with potential new entrants into the market.
Learn More about the AI Fund
We are seeing strong interest in this fund as prior AI Fund vintages were oversubscribed, and we’ve had to establish a waitlist to accommodate interest.
If interested, we recommend securing a spot promptly.
Max Accredited Investor Limit: 249
Discovery and Document Review
It’s been more than ten years since the American College of Trial Lawyers suggested that the present model of civil discovery in the United States is “broken,” and calls for major reform have only continued since then. Against this backdrop, I fully expect AI to profoundly disrupt the current regime of document review and discovery. To this I will add that the widely held view among trial lawyers is that change is sorely needed.
The current practice of staffing teams of contract attorneys and junior associates to sift through gigabytes of e-mails, corporate records, and employee note files must end. It is wildly inefficient and enormously expensive. The costs involved with civil discovery in even moderately complex litigation – both in terms of money and employee time – can heavily influence a claim’s chances of success or failure. The burdens of searching for, reviewing, and producing voluminous documents looms large over settlement negotiations for defendants. These expenses distort the settlement process, influencing the resolution of claims as a cost-benefit proposition, rather than on their merits.[1] Plaintiff attorneys task associates with spending days, weeks, or longer sifting through those productions, to sort relevant from irrelevant, and the “hot docs” from the only marginally significant. The costs of this process can limit what types of claims find qualified representation.
The current approach is built on today’s ability for AI to solve Well-Defined Problems. Does a note file contain a search term or not? Is an email within a date range for production or not? But as the capabilities of AI expand to include tasks that more closely approach Ill-Defined Problems, law firms will increasingly be able to automate the process of identifying documents that substantively deal with relevant subject matter, instead of conducting a binary check for the presence or absence of keywords. One of most popular uses of AI is to summarize long articles, which can be adapted to summaries of lengthy depositions, expert reports, and more. AI may even be able to parse the substance of relevant documents to flag favorable or unfavorable evidence. This should allow e-discovery to become more narrowly targeted at discoverable evidence and eliminate much of the cost involved with secondary review by contract attorneys.
If this were not enough, it’s likely that secondary payors of discovery expenses – litigation financiers, some types of investors, and especially insurers – will begin to exert significant pressure on litigants and attorneys to make sure of cost-effective AI solutions to documents review and production. Particularly insurance companies, which can and do limit the expenses that an insured can pay with policy assets, may limit their roster of approved law firms to those that have embraced AI-driven discovery or refuse to pay for the hourly costs of human document reviewers.
[1] See, e.g., Pension Benefit Guar. Corp. v. Morgan Stanley Invt. Mgmt., 712 F.3d 705, 719 (2d Cir. 2013) (“[T]he prospect of discovery in a suit claiming breach of fiduciary duty is ominous, potentially exposing the ERISA fiduciary to probing and costly inquiries and document requests . . . . This burden, though sometimes appropriate, elevates the possibility that a plaintiff with a largely groundless claim [will] simply take up the time of a number of other people, with right to do so representing an in terrorem increment of the settlement value, rather than a reasonably founded hope that the discovery process will reveal relevant evidence.”).
However, as the scope of the issues to be considered widens and the inputs required to arrive at an answer grow more ambiguous and rely on implications and inferences one or more steps removed from the “plain meaning” of authoritative texts, I expect that AI will struggle. Natural language processing alone will not answer questions like “How does the entwinement doctrine apply to viewpoint-based moderation by major social media platforms?” or “How will the Major Questions Doctrine be delimited and applied by the current Supreme Court?” or “Does the substantive due process right to family integrity allow a civil rights claim in the case of misconduct by child protective services?”
Moreover, in my experience, the current crop of AI tools seems to struggle to distinguish high-quality inputs from low-quality inputs without fairly specific training aligned with a user’s preferences. Someone could probably invest the time needed to train an AI on their preferences regarding use of law review articles, industry journals, treatises from the 20th century (or before), dissenting opinions, unpublished decisions, and other inputs that can vary widely in their persuasive value. However, in my own practice, the value of these sources changes very significantly from issue to issue, compounding the difficulty in providing general training to an AI to weigh these authorities when conducting analysis. Also, training an AI requires a more technical skillset than many attorneys have. Additionally, this investment of time could undermine the value proposition of AI as a time-saver in the first place.
Drafting Pleadings and Briefs
On this issue, some might say, “The future is already here,” but is it? AI is already being used to put together pleadings, motions, and other filings, but it has significant limits that will likely be with us for a while. First and foremost, Chat-GPT can already put together a sometimes-passable complaint or brief. But is this really what a committed advocate wants to submit?
Attorneys are expected to be zealous advocates for their clients, and to handle each matter competently. I would not want to turn in merely passable work on behalf of my clients, and I believe most, if not all, of my contemporaries feel the same way. Some users of AI drafting tools describe their output as “superficially impressive” but often lacking a connection to the goal of the document being drafted. Whether that is a lock-up agreement that includes terms contrary to its purpose, a brief citing nonexistent case law, or a buy/sell contract missing essential terms, there are plenty of stories of generative AI not yet being up to the task of putting together sophisticated legal documents.
If more were needed, courts are increasingly ordering litigants to certify that they’ve had a human being review any portion of a filing drafted by AI for accuracy. To be sure, this would be good practice in the absence of such an order, but increasingly litigants are expressly prohibited from excessively relying on AI tools to devise court filings. Beyond this, some local rules are highly prescriptive with respect to the format and content of certain filings, such as summary judgment briefing, and failure to follow those rules can mean serious penalties for a litigant. I am highly skeptical that AI will be able to reliably produce drafts that can navigate these procedural hurdles anytime soon.
Thus, I think the most that can be said of AI at this point is that it can sometimes be a serviceable first step in quickly putting together a rough draft of some basic legal documents, like pleadings. But even here, I would keep a human’s hands firmly on the steering wheel for the foreseeable future.
Trial Advocacy
Here, we are still long way away from robot trial lawyers, despite some faltering early attempts. Until we have a physical manifestation of an AI that can deliver opening and closing statements, present exhibits, address the court, make eye contact with jurors, and argue evidentiary issues, I don’t think today’s trial attorneys are in any danger of being automated out of a job.
Furthermore, there are an almost infinite number of choices and decisions to be made in terms of how to present a case at trial. From the sequencing of exhibits and witness testimony, to the scope of a cross-examination, to which Motions in Limine to pursue and which to leave for the heat of the moment, all the way down to your client’s outfit on the stand, trial advocacy revolves around the kinds of Ill-Defined Problems that AI doesn’t seem ready to take on.
Transactional
Like litigation, transactional work incorporates Well-Defined and Ill-Defined Problems. And like litigation, transactional work is susceptible to AI disruption to the extent it incorporates the former, but will likely resist disruption to the degree it involves the latter. In addition, transactional work must navigate subjectively human considerations such as fairness, risk-reward balancing, and maintaining long-term collaborative relationships. To somewhat oversimplify the stages of a transactional deal, the role of AI can be described in the following phases in the lifecycle of a deal.
Negotiation
As with trial advocacy, I expect the subjective and uniquely human elements of transactional negotiation to be insulated from disruption by AI for the foreseeable future. It’s possible AI could be used to illuminate industry baselines as starting positions, like the prevailing EBITDA multiples for a corporate acquisition or the market rates for equity as a component of executive compensation. But the actual back-and-forth in negotiation is too dependent on “EQ” for software that predicts linguistic patterns to be helpful.
Moreover, deal structuring is the type of Ill-Defined Problem that incorporates too many inputs for AI to handle. The best deals often require a degree of creativity that I don’t think AI can match. Choosing to structure a payment as an earn-out or installments with a holdback, whether to pay cash or stock, and even something as simple as the name of the surviving corporation in a merger of equals are all problems that the current generation of AI wasn’t built to solve.
Deal Documents
I expect there will be a large, disruptive role for AI to play in drafting deal documents, but only in the early stages. As any veteran of financing, acquisition, or other complex deals will tell you, the process of putting together documents begins by identifying the right “form,” or transactional document previously used in a similar deal, to adapt to the present transaction. This is often a matter of locating the most recently used document for the same type of transaction in the same jurisdiction, identifying what sections apply, and what sections need to be changed to reflect the particular terms of the current deal.
This first step bears some resemblance to legal research in support of litigation. It involves sifting through a library of documents to find the ones that include language that’s relevant to a current issue. Whether the search is for case law or asset purchase agreements, it’s susceptible to disruption by AI. Training an AI on a library of deal documents can enable it to use natural language processing capabilities to quickly identify another set of deal documents that can be adapted to a similar transaction. Indeed, a properly trained AI could cobble together a starting point for deal documents that pulls the most applicable sections from several disparate “form” agreements, reducing the time to put together a first draft agreement from hours to minutes.
Over time, an AI might even be trained to create first drafts that are tailored to the parameters of a given deal, but given the limitations on AI legal drafting mentioned above, I wouldn’t allow AI to completely control the process. Remember, AI’s principal ability is to parse natural language and extrapolate what comes next from pattern recognition. AI doesn’t have any contextual understanding of the documents it’s drafting or the purpose they’re meant to serve. Its ability to pursue the unique goals of a particular deal will likely be limited.
Regulatory Considerations
AI will have a role to play in identifying and, to a lesser degree, addressing regulatory compliance issues that may come up in the context of a transaction. But I would definitely still have a human piloting the flight through regulatory territory.
Issue-spotting regulatory issues can sometimes pose Well-Defined Problems. Identifying when a capitalization table is getting big enough to require share class registration under the Securities Exchange Act, when a total number of consumers subjects a business to certain state data privacy statutes when a manufacturing process implicates the Toxic Substances Control Act, and so on, requires fairly straightforward application of mathematical data sets to an objective legal standard.
But other times, regulatory issues can be more subjective and hinge on the nuanced, non-semantic meaning of regulatory language. I don’t think an AI will be able to tell a lawyer if marketing materials are fair and balanced, when a data security program is reasonably designed, or when a business practice is unscrupulous or oppressive. Even if an AI could understand these concepts, regulatory standards based on the same language evolve over time and across different regulatory administrations – the same words can come to mean different things. In this regard, I don’t think any AI program can substitute for the judgment of an experienced attorney who can anticipate the human predispositions of a regulator. AI can’t provide the intuitive sense of fairness and morality that sometimes influences a regulator’s approach.
Finally, what to do when a regulatory issue arises can, and usually does, raise broad and thorny questions that are probably beyond the ability of AI to answer. Exactly how explicit should a Form 8-K filing be in describing an unscheduled material event? What should an employer’s position statement say when confronted with an EEOC charge that it maintained a hostile working environment? How do parties to a merger agreement best make the case that their combination will not substantially lessen competition or create a monopoly? The issues at work in any of these scenarios are too subjective and too far-ranging for AI to handle.
A Final Cautionary Note
Lastly, beware of products that emphasize their use of AI, but which offer minimal value. For example, I was pitched on a number of AI contract services that would summarize the choice of law or generate a table of contents showing me the pages on which various sections of the contract appear. A well-drafted choice of law paragraph is usually about three sentences long. My existing Find-and-Replace command in my word processing program can locate any section of a contract I might be interested in with a few keystrokes. I was deeply skeptical that these services were worth the fees they charged.
In short, just as many businesses marketed themselves in terms of “the blockchain” when that was popular, watch out for products describing themselves as “AI” but adding little value today.
Looking Beyond
The general, far-ranging precepts I’ve discussed should inform how AI will intersect with some of the narrower subspecialties in the practice of law, as well. Fields that heavily involve the adjustment and reformulation of template documents — such as trusts and estates, some areas of taxation, and perhaps some areas of IP prosecution — will likely look quite different in five years. On the other hand, fields that involve nuance, subjectivity, formulation of complex strategy, or close interfacing with human actors will see less change. These include white-collar defense and investigation, antitrust, major appellate advocacy, and some facets of labor and employment law.
Aside from which areas of practice are susceptible to disruption, the effect of AI will vary depending on attorney seniority. Senior attorneys making broad, high-level decisions or choosing tactical, strategic direction for a wide-ranging engagement probably don’t need to worry. Junior attorneys responsible for collecting and processing information and conducting low-level analysis probably do.
In some cases, incumbents may decide to adopt and integrate AI into their service offerings. You can already see this at work with some of the major legal research services. But the truly seismic changes will likely come from outsiders not invested in an existing service model, not obligated to recover costs incurred in paying large numbers of human employees, and who will have more freedom to reconceptualize new solutions to “traditional” legal problems.
As we enter the AI age, keep your eye on three more wild cards that will heavily influence the role of AI in legal sphere. First, state-level regulation of the practice of law and the Rules of Professional Conduct could limit the use cases for AI. Indeed, we’ve already seen some state regulators shut down a fledging attempt to launch an entirely automated legal practice. The understandable inclination of professional associations to protect their own may lead to bar associations lobbying law enforcement to adopt an aggressive stance against marketing AI as a substitute, rather than a supplement, for a lawyer billing by the hour.
Second, as AI makes inroads into the legal profession, there will be inevitable missteps and shortcomings. In some cases, these will lead to malpractice litigation that will create standards of care further shaping the relationship between lawyer, client, and AI.
Third is the looming possibility of copyright infringement litigation against AI services trained on copyrighted works. We don’t need AI-powered research to remind us that a copyright holder has enforceable rights against certain derivative works. So what happens when an AI is trained on copyrighted works? What about when AI is designed to produce works similar to existing copyrighted content? Some of these issues are already the subject of pending litigation, which could profoundly affect the way AI is designed and operates.
Conclusion
The unknown future rolls toward us. But, if a machine – a GPT – can learn the tricks of the legal trade, maybe we lawyers can learn to master AI, too.
Of course, this blog wouldn’t be the work of an attorney if it did not include a disclaimer.
This publication is for informational purposes only, is not personalized advice, is not a solicitation or call to action to engage in any transaction, does not create an attorney-client relationship, and is not necessarily representative of the views of Alumni Ventures, LLC and its affiliates, or any other client or employer of the author.